As cosmic rays crash into the Earth’s atmosphere, air showers containing atmospheric muons and neutrinos are produced. The atmospheric neutrinos are then detected by DeepCore, a denser array of sensors in the center of the IceCube detector at the South Pole. Compared to the main IceCube detector, DeepCore is sensitive to neutrinos down to energies of only a few GeV, or a 100 times lower in energy.
On the way to IceCube, atmospheric neutrinos travel sufficient distances to allow transformations into different types or “flavors”—electron, muon, and tau—in a phenomenon called neutrino oscillation. These oscillations occur as a result of the “mixing” between neutrino mass and different flavor states, but the detection of atmospheric neutrino oscillations can be difficult.
In a study recently submitted to Physical Review Letters, the IceCube Collaboration presents an improved measurement of neutrino oscillation parameters using the largest atmospheric dataset of over 150,000 candidate neutrino events between 5 GeV and 100 GeV and an advanced reconstruction method based on convolutional neural networks (CNN). The results are the most precise to date using atmospheric neutrinos.
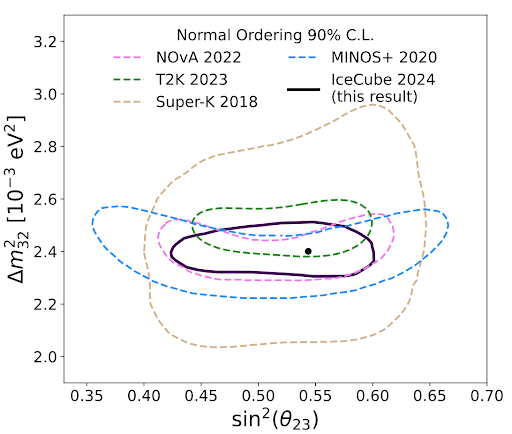
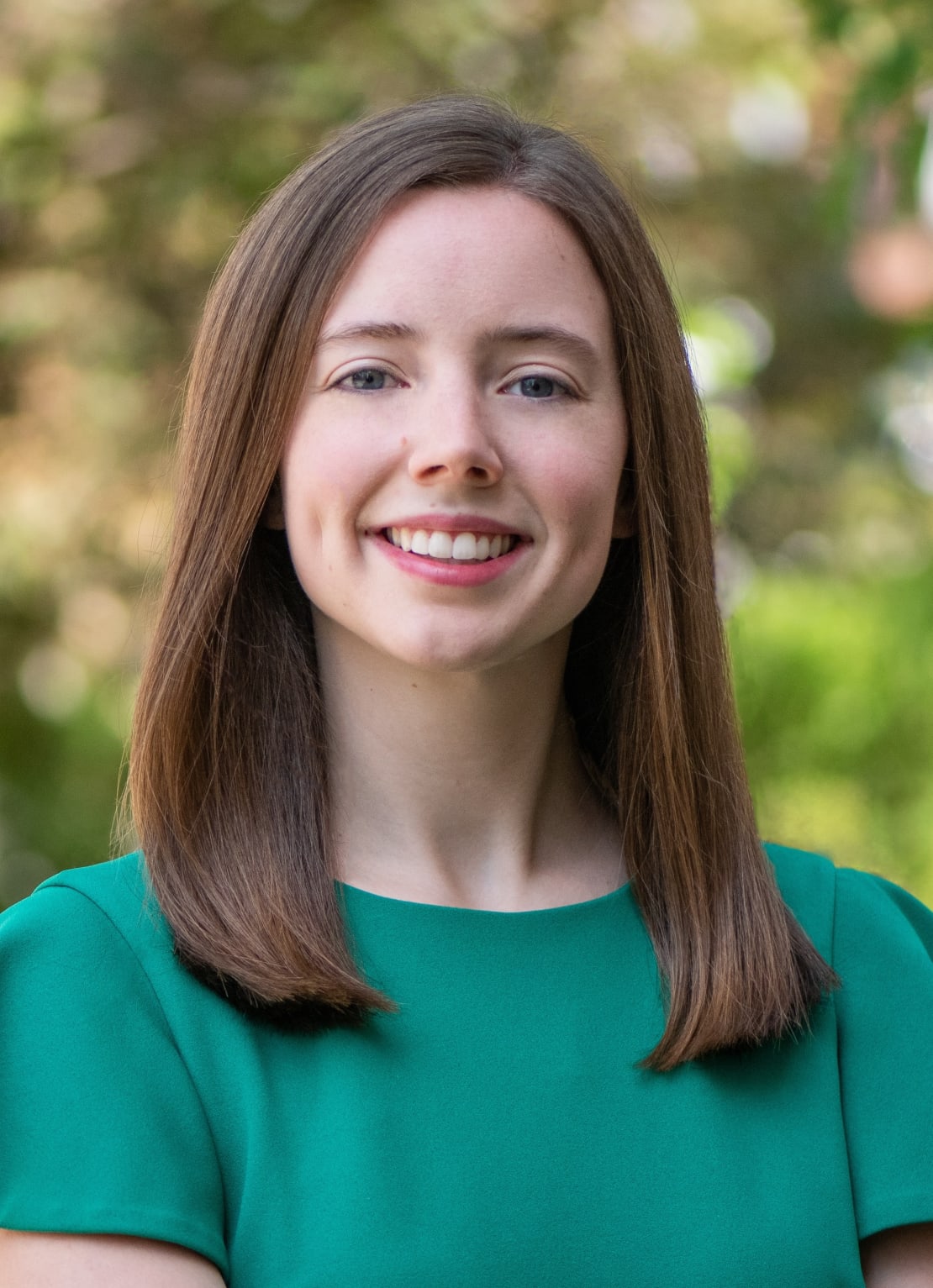
The study utilized DeepCore data taken between 2012-2021 that included candidate neutrino events with reconstructed energies.
“This is the first time we have leveraged the full statistical power from IceCube DeepCore’s first decade of data,” says Kayla DeHolton, a postdoctoral researcher at Pennsylvania State University who contributed to the work. “In doing so, we have curated one of the largest GeV neutrino samples in the world, while maintaining greater than 99% neutrino purity. ”
“We also carefully studied the systematic uncertainties that arise from our imperfect knowledge of our models and chose some to use as free nuisance parameters that fit together with the physics parameters for our data,” says Shiqi Yu, a research assistant professor at the University of Utah and former postdoctoral researcher at Michigan State University (MSU), where she conducted the work. Yu is a co-lead of the study along with Jessie Micallef, who conducted the work as a PhD student at MSU. Micallef is now a postdoctoral fellow at the National Science Foundation’s AI Institute for Artificial Intelligence and Fundamental Interactions.
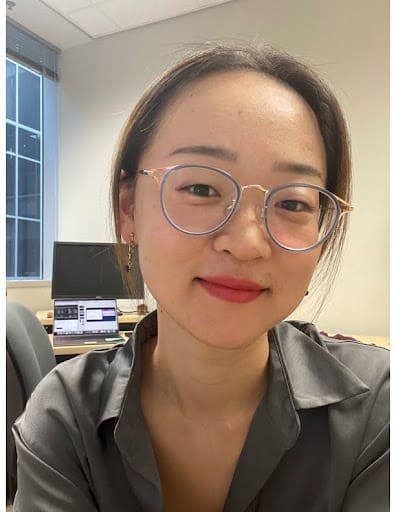
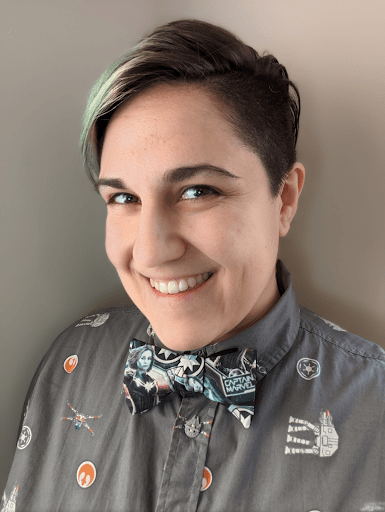
Using CNNs, which use three-dimensional data for image classification, Micallef and Yu first developed use cases for the CNNs to focus on the DeepCore region and trained them to reconstruct different properties of particle interactions in the detector. They then used the CNN reconstructions to select qualified neutrino interactions that happened in or near the DeepCore region to produce a neutrino-dominated dataset with well-reconstructed energies and zenith angles.
“Harnessing the power of machine learning for full neutrino event reconstruction is an exciting advancement for these analyses, as we are starting to contend with larger datasets and future detector upgrades,” says Micallef.
The CNN-reconstructed analysis-level dataset yielded increased signal efficiency and background suppression, resulting in a measurement of neutrino oscillation parameters, ∆m232 and sin2(θ23), with both a seven-fold increase in statistics compared to previous DeepCore oscillation results and high neutrino purity. Furthermore, the results are consistent with measurements from other neutrino detectors including long-baseline accelerator experiments.
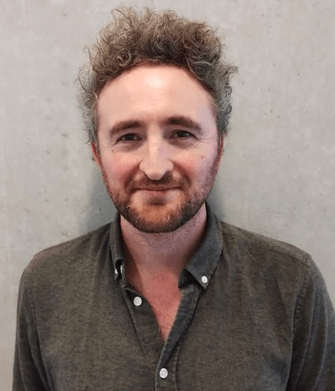
“The very high statistics of this measurement means that accurate modeling of systematic uncertainties related to the IceCube detector, neutrino flux and interactions in the ice are more important than ever,” says Tom Stuttard, a research assistant professor at the Niels Bohr Institute who also contributed to the work. “The tools to make this analysis possible have been built up by an international group effort over the last few years, and it is exciting to finally reach comparable precision to dedicated accelerator experiments with IceCube, an experiment that was not constructed with neutrino oscillation measurements in mind.”
Yu notes that the CNN-reconstructed analysis-level dataset is already being used for other neutrino oscillation analyses, such as determining the neutrino mass ordering and non-standard neutrino interactions and for atmospheric tau neutrino appearance analyses.
“The atmospheric neutrino dataset from DeepCore exhibits relatively high energies in the oscillation analyses, which is unique compared to existing accelerator-based experiments,” says Yu. “Given our dataset and independent analysis, it is interesting to see agreement and consistency in physics parameter measurements.”
In 2025-2026, IceCube will deploy the Upgrade project consisting of seven additional strings to be placed within the DeepCore region. These densely-spaced strings will feature new sensor configurations, such as modules with multiple sensors. The IceCube Upgrade, in combination with the existing IceCube DeepCore, will increase the sample size and the amount of information collected, greatly enhancing IceCube’s capabilities in the GeV region.
Adds Micallef, “This reinforces the benefit of using machine learning reconstructions for these neutrino samples.”
+ info “Measurement of atmospheric neutrino oscillation parameters using convolutional neural networks with 9.3 years of data in IceCube DeepCore,” IceCube Collaboration: R. Abbasi et al. Submitted to Physical Review Letters. arxiv.org/abs/2405.02163