The IceCube Collaboration, in conjunction with Kaggle, the Technical University of Munich (TUM), Munich Data Science Institute (MDSI), the Collaborative Research Center SFB 1258, Excellence Cluster ORIGINS, and PUNCH4NFDI, announces the launch of the IceCube – Neutrinos in Deep Ice project. This outreach project invites everyone to present a machine learning solution that tackles the problem of IceCube event reconstruction.
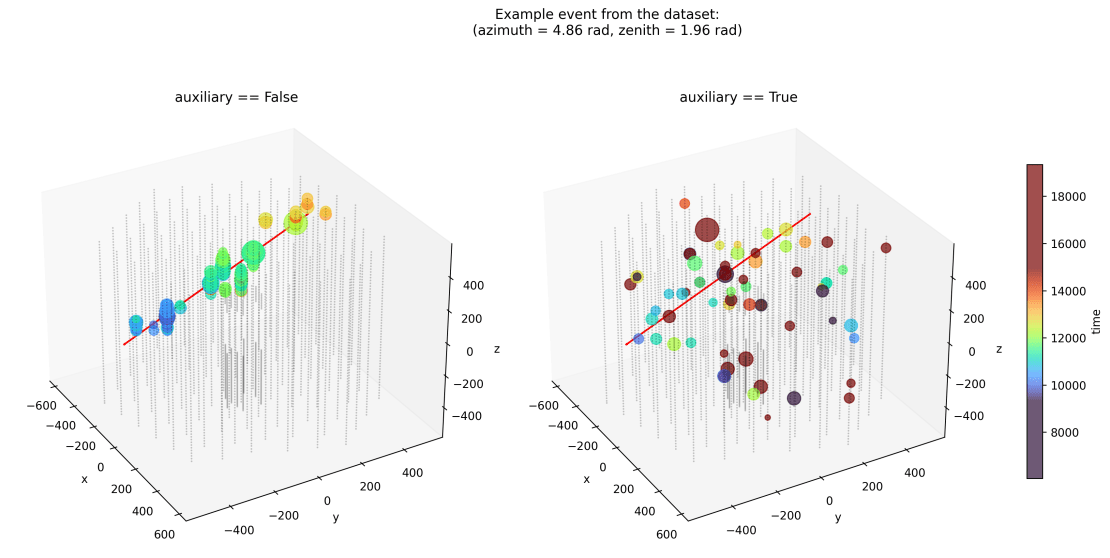
The IceCube Neutrino Observatory, which encompasses a cubic kilometer of Antarctic ice, searches for nearly massless particles called neutrinos in order to better understand the cosmos. Scientists achieve this by estimating the direction of neutrino events, allowing them to trace neutrinos back to their sources. However, with an average of three thousand events per second to process, the stream of data becomes daunting using current, traditional methods. This is where machine learning can shine.
Over the next three months, participants will be challenged to devise a solution that can quickly and accurately process a large number of events to identify the direction that neutrinos came from. Their solutions will be scored on a hidden test set of one million events, and the three teams with the best overall performance on these events will win prize money.
Participants (excluding IceCube and Technical University of Munich members) will have a chance to win a total of $50k in cash prizes.
“First and foremost, I hope that this competition will allow participants to learn about the wonderful world of neutrinos, our fantastic detector, and to think about the problem of event reconstruction,” says Philipp Eller, IceCube member and postdoctoral associate at the Technical University of Munich in Germany, who is organizing the competition. “My sincere hope is that we will end up with improved methods to do our science.”
The final submission deadline is April 20, 2023.